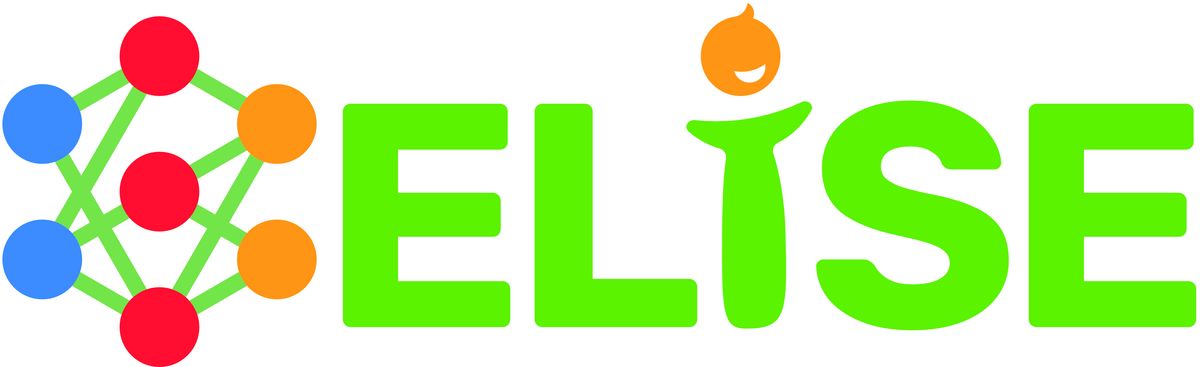
A learning and interoperable, smart clinical decision support system for the paediatric intensive care
ELISE aimed at developing a digital clinical decision support system (CDSS) for the paediatric intensive care unit to optimize diagnostic and therapeutic routine processes. Especially the early identification and anticipation of life-threatening diagnoses are crucial because the paediatric intensive care setting is a complex knowledge- and experience-based area that continuously challenges healthcare professionals. All diagnostic and therapeutic measures are shaped by highly individual variations due to the age-specific development stages of children and the heterogeneous, partly seldom, diseases within this patient population. To address these challenges, we develop ELISE, which can support clinicians in the early identification of Systemic Inflammatory Response Syndrome (SIRS), sepsis, and associated organ dysfunctions (i.e., hepatic/ hematologic/ respiratory/ renal/ cardiovascular organ dysfunction).
The ELISE CDSS consists of multiple, target-condition-specific knowledge-based detection and data-driven prediction models. These models are developed and evaluated for their diagnostic accuracy (i.e., measured by sensitivity and specificity) before they are validated. Upon achieving an acceptably high level of accuracy to detect and/or predict the presence of diagnostic events, these models may be integrated in a routine application of the CDSS used in the paediatric intensive care.
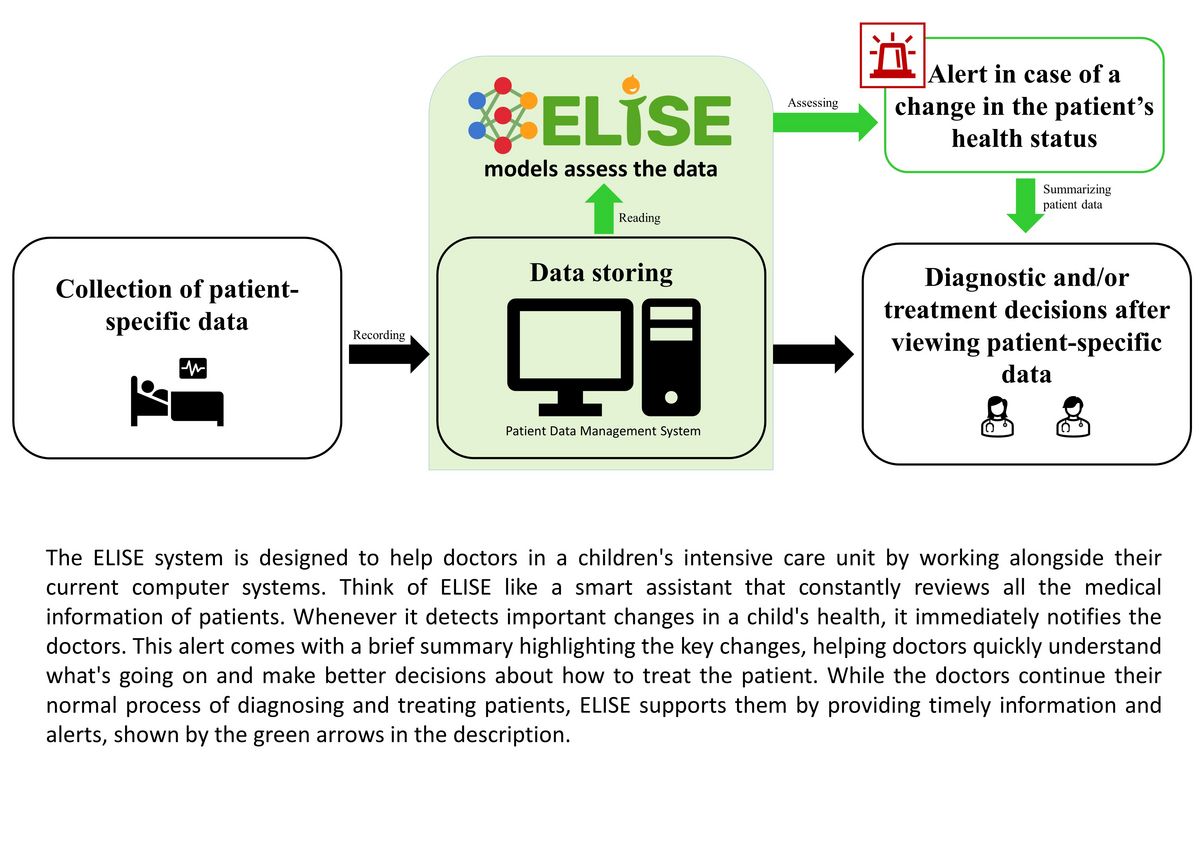
Project overview
Contact | Julia Böhnke, MSc; Nicole Rübsamen, PhD |
Project coordinator at IESM | Univ.-Prof. Dr. med. André Karch, MSc |
Funding |
German Federal Ministry of Health Funding reference: 2520DAT66C Funding period: October 2020 – December 2023 (Analyses are still ongoing) |
Cooperation partner |
Bioinformatics and Digital Health, Fraunhofer ITEM (data-driven prediction model developer) Medisite GmbH (software developer) Paediatric Cardiology and Paediatric Intensive Care, Hannover Medical School (clinicians and data providers) Institute for Medical Informatics, Peter L. Reichertz Institute (knowledge-based detection model and data-driven prediction model developer) Institute of Epidemiology and Social Medicine, University Münster (epidemiologists) |
Awards
- Lower Saxony Health Award 2021 (https://digitalagentur-niedersachsen.de/gewinner-niedersaechsischer-gesundheitspreis-2021/)
Publications
- Das, P., Wiese, L., Mast, M., Boehnke, J., Wulff, A., Marschollek, M., Bode, L., Rathert, H., Jack, T., Schamer, S., Beerbaum, P., Rübsamen, N., Karch, A., Groszweski-Anders, C., Haller, A., & Frank, T. (2024). An attention-based bidirectional LSTM-CNN architecture for the early prediction of sepsis. International Journal of Data Science and Analytics, 1–15. https://doi.org/10.1007/s41060-024-00568-z
- Böhnke, J., Zapf, A., Kramer, K., Weber, P., ELISE Study Group, Karch, A., & Rübsamen, N. (2024). Diagnostic test accuracy in longitudinal study settings: theoretical approaches with use cases from clinical practice. Journal of clinical epidemiology, 169, 111314. Advance online publication. https://doi.org/10.1016/j.jclinepi.2024.111314
- Das, P. P., Mast, M., Wiese, L., Jack, T., & Wulff, A. (2024). Algorithmic Fairness in Healthcare Data with Weighted Loss and Adversarial Learning. In Intelligent Systems and Application, ed. Kohei Arai. Cham: Springer Nature Switzerland, 264-83. https://doi.org/10.1007/978-3-031-47715-7_18
- Bode, L., Mast, M., Rathert, H., Elise Study Group, Jack, T., & Wulff, A. (2023). Achieving Interoperable Datasets in Pediatrics: A Data Integration Approach. Studies in health technology and informatics, 305, 327–330. https://doi.org/10.3233/SHTI230496
- Tute, E., Mast, M., & Wulff, A. (2023). Targeted Data Quality Analysis for a Clinical Decision Support System for SIRS Detection in Critically Ill Pediatric Patients. Methods of information in medicine, 62(S 01), e1–e9. https://doi.org/10.1055/s-0042-1760238
- Das, P. P., Wiese, L., and ELISE Study Group. (2023). Explainability Based on Feature Importance for Better Comprehension of Machine Learning in Healthcare. In New trends in Database and Information Systems, eds. Alberto Abelló, Panos Vassiliadis, Oscar Romero, Robert Wrembel, Francesca Bugiotti, Johann Gamper, Genoveva Vargas Solar, and Ester Zumpano. Cham: Springer Nature Switzerland, 324–35. https://doi.org/10.1007/978-3-031-42941-5_28
- Wulff, A., Bode, L., Mast. M. (2022), Ein wissensbasiertes, interoperables Entscheidungsunterstützungssystem für die pädiatrische Intensivmedizin. mdi medizin://dokumentation/informatik/informationsmanagement/. 2022;3:85-87. German.
- Böhnke, J., Rübsamen, N., Mast, M., Rathert, H., ELISE Study Group, Karch, A., Jack, T., & Wulff, A. (2022). Prediction models for SIRS, sepsis and associated organ dysfunctions in paediatric intensive care: study protocol for a diagnostic test accuracy study. BMJ paediatrics open, 6(1), e001618. https://doi.org/10.1136/bmjpo-2022-001618
- Wulff, A., Mast, M., Bode, L., Rathert, H., Jack, T., & Elise Study Group (2022). Towards an Evolutionary Open Pediatric Intensive Care Dataset in the ELISE Project. Studies in health technology and informatics, 295, 100–103. https://doi.org/10.3233/SHTI220670
- Böhnke, J., Varghese, J., ELISE Study Group, Karch, A., & Rübsamen, N. (2022). Systematic review identifies deficiencies in reporting of diagnostic test accuracy among clinical decision support systems. Journal of clinical epidemiology, 151, 171–184. https://doi.org/10.1016/j.jclinepi.2022.08.003
- Wulff, A., Mast, M., Bode, L., Marschollek, M., Schamer, S., Beerbaum, P., Rübsamen, N., Böhnke, J., Karch, A., Das, P. P., Wiese, L., Groszewski-Anders, C., Haller, A., Frank, T., Rathert, H., & Jack, T. (2022). ELISE - An open pediatric intensive care data set [Data set]. https://leopard.tu-braunschweig.de/receive/dbbs_mods_00070468
- Bode, L., Schamer, S., Böhnke, J., Bott, O. J., Marschollek, M., Jack, T., Wulff, A., & ELISE Study Group (2022). Tracing the Progression of Sepsis in Critically Ill Children: Clinical Decision Support for Detection of Hematologic Dysfunction. Applied clinical informatics, 13(5), 1002–1014. https://doi.org/10.1055/a-1950-9637
- Mast, M., Marschollek, M., Jack, T., Wulff, A., & Elise Study Group (2022). Developing a Data Driven Approach for Early Detection of SIRS in Pediatric Intensive Care Using Automatically Labeled Training Data. Studies in health technology and informatics, 289, 228–231. https://doi.org/10.3233/SHTI210901
- ly Detection of SIRS in Pediatric Intensive Care Using Automatically Labeled Training Data. Studies in health technology and informatics, 289, 228–231. https://doi.org/10.3233/SHTI210901
- Wachenbrunner J, Mast M, Böhnke J, Bode L, Rübsamen N, Rathert H, et al. Developing a Complex Rule-Based Clinical Decision Support System for Detection of Acute Kidney Injury after Pediatric Cardiac Surgery. In: The Thoracic and Cardiovascular Surgeon [Internet]. Georg Thieme Verlag KG; 2024 [cited 2024 Feb 26]. p. DGPK-V04. Available from: http://www.thieme-connect.de/DOI/DOI?10.1055/s-0044-1780717
- Böhnke J, Zapf A, Kramer K, Weber P, Karch A, Rübsamen N. Methods for estimation of diagnostic test accuracy using longitudinal data. In German Medical Science GMS Publishing House; 2023. p. DocAbstr. 162. Available from: https://www.egms.de/static/en/meetings/gmds2023/23gmds056.shtml
- Das PP, Mast M, Wiese L, Jack T, Wulf A. Data Extraction for Associative Classification using Mined Rules in Pediatric Intensive Care Data. In Gesellschaft für Informatik e.V.; 2023 [cited 2024 Apr 26]. p. 981–94. Available from: https://dl.gi.de/handle/20.500.12116/40376
- Jack, T., Wulff, A., Rathert, H., Montag, S., Marschollek, M., & Beerbaum, P. (2022). Development of a Clinical Decision Support System for the Detection of SIRS after Surgery for Congenital Heart Disease. The Thoracic and Cardiovascular Surgeon, 70(S 2), DGPK-V36. https://doi.org/10.1055/s-0042-1742994
- Böhnke J, Varghese J, Karch A, Rübsamen N. Reporting quality and risk of bias in studies evaluating the diagnostic test accuracy of clinical decision support systems: a systematic review of current practice. In German Medical Science GMS Publishing House; 2022. p. DocAbstr. 81. Available from: https://www.egms.de/static/en/meetings/gmds2022/22gmds006.shtml
Websites (extern)
- https://www.bundesgesundheitsministerium.de/ministerium/ressortforschung-1/handlungsfelder/forschungsschwerpunkte/digitale-innovation/modul-3-smarte-algorithmen-und-expertensysteme/elise.html
- https://plri.de/forschung/projekte/elise